AI face swap technology has become a popular tool for creating engaging and entertaining content. By using sophisticated algorithms, this technology allows users to swap faces in photos and videos easily and quickly. These tools enable the production of realistic or humorous images, making them ideal for memes and social media posts.
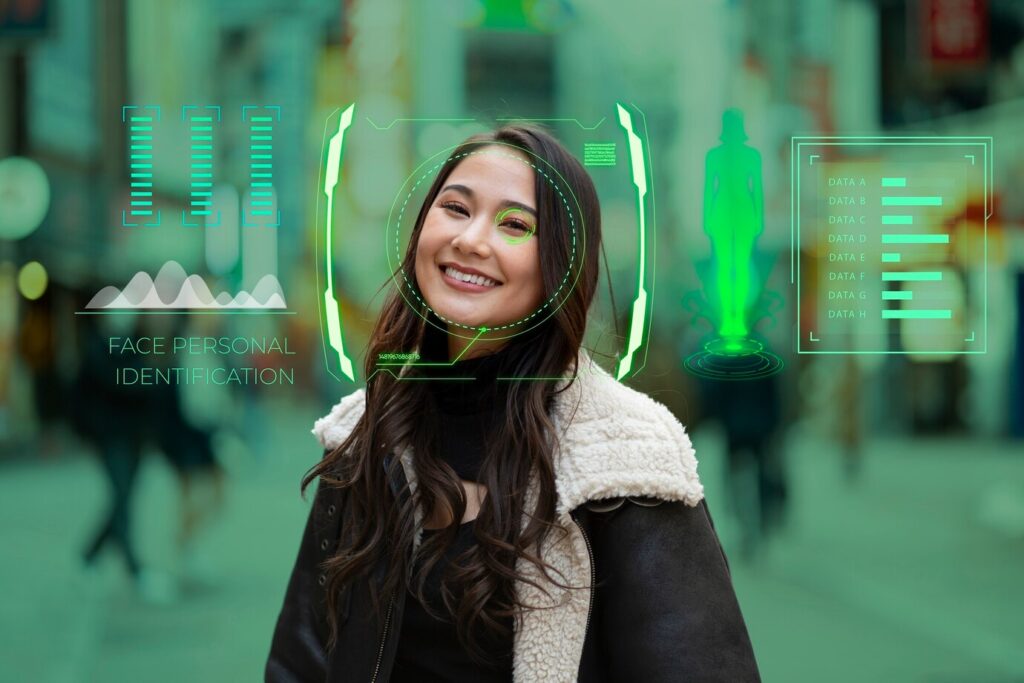
As more people explore this technology, its applications continue to grow. From creative projects to marketing campaigns, AI face swaps offer a unique way to capture attention. However, users must also consider the ethical implications and potential challenges that come with manipulating images of individuals.
Understanding how to use AI face swap tools effectively and responsibly is essential. This article will explain the technology behind it, explore its many uses, and discuss important considerations for anyone looking to dive into this trending phenomenon.
Key Takeaways
- AI face swap technology uses advanced algorithms for realistic image manipulation.
- Consider the ethical implications before creating AI face swaps.
- Responsible use can lead to creative and engaging content for various audiences.
Understanding AI Face Swap Technology
AI face swap technology allows users to exchange faces in images and videos using advanced processes. This involves a few key principles, techniques, and algorithms that enable realistic results.
Basic Principles of Face Swapping
At its core, face swapping requires the identification of facial features. The process begins with selecting a base image and a target image. The software analyzes key facial points, like eyes, nose, and mouth.
Using these reference points, the technology aligns the facial features from the second image onto the first. After alignment, the colors and shapes are adjusted to create a seamless blend. This careful analysis is vital for achieving a realistic swap.
Deep Learning Algorithms in Face Swapping
Deep learning plays a crucial role in improving the accuracy of face swaps. These algorithms train models on large datasets of images to recognize and replicate various facial attributes.
The training process helps the model learn how to extract important features from faces. By analyzing numerous images, it becomes adept at understanding expressions, angles, and lighting conditions. This knowledge allows for more convincing swaps.
Through continuous training, these models refine their output, resulting in less noticeable differences between the swapped face and the original. This tech helps produce high-quality images that can fool the naked eye.
Roles of Generative Adversarial Networks (GANs)
Generative Adversarial Networks (GANs) are a powerful tool in face swapping. GANs consist of two neural networks: a generator and a discriminator.
The generator creates fake images, while the discriminator evaluates them against real images. The two networks work together in a competitive process. This leads to improved quality and realism in face swaps.
As the generator improves, it learns to create more lifelike faces. The discriminator becomes better at spotting fakes, pushing the generator to create even more realistic images. This cycle enhances the overall effectiveness of AI face swapping technology.